Machine learning in retail: essentials and 10 key applications – TechNative
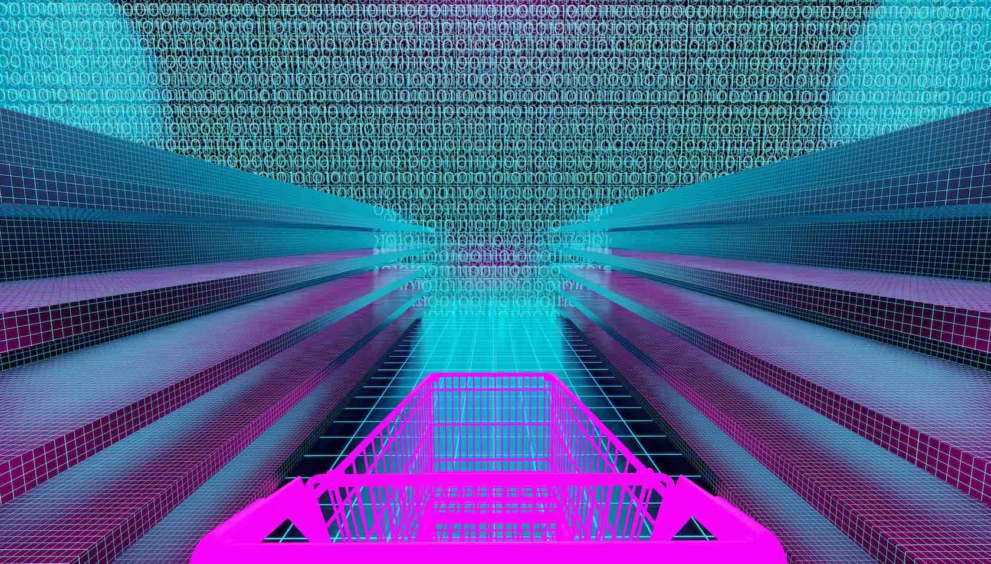
In the eCommerce landscape, Artificial Intelligence (AI) is reshaping the game. This blog series explores how AI’s intelligent algorithms are revolutionizing online businesses, from personalized product recommendations to efficient inventory management. Join us for insights on leveraging AI to enhance the digital shopping experience and overall success in eCommerce.
In recent years, between lockdowns, curfews, supply chain disruptions, and energy crunches, retailers must have felt like dinosaurs trying to dodge a rain of asteroids and avoid extinction.
But unlike those giant prehistoric reptiles, the retail industry could count on a full array of technological innovations to better meet the challenges of these difficult times.
One of the most impactful tools in this arsenal has certainly turned out to be artificial intelligence, including its powerful sub-branch known as machine learning (ML). Let’s briefly frame the nature of this technology and explore the key use cases of machine learning in retail.
The role of machine learning in retail
Machine learning in retail relies on self-improving computer algorithms created to process data, spot recurring patterns and anomalies among variables, and autonomously learn how such relations affect or determine the industry’s trends, phenomena, and business scenarios.
The self-learning and contextual understanding potential of machine learning systems can be harnessed in retail to:
10 machine learning use cases that redefine retail
How can retailers benefit from the aforementioned capabilities of ML algorithms on the ground? Here are some of the most relevant machine learning use cases in a typical retail scenario.
1. Targeted ads
While mostly used in ecommerce, targeted marketing represents a powerful tool to route potential customers both towards online platforms and traditional stores. This involves segmenting users based on an ensemble of behavioral, psychographic, demographic and geographic parameters (such as their purchase and browsing history, age, gender, interests, region, etc.) and targeting them with fully personalized ads and promotions.
2. Contextual shopping
A different, more interactive solution to catch users’ attention and lead them towards your ecommerce platform is contextual shopping. This marketing tool taps into machine learning and computer vision to identify and point out the merchandise shown in videos and pics on social media while offering a “shortcut” to reach the related product page in an online shop.
3. Recommender engines
Once users land on an online platform, they may feel lost among a massive selection of merchandise. Recommendation engines are powerful tools designed to drive customers towards the products they may actually need.
To provide tailored suggestions, these systems can adopt a content-based filtering approach, namely recommend items with similar features to those purchased in the past, or opt for collaborative filtering, which implies suggesting products ordered by other customers with similar purchase patterns, personal traits, and interests.
4. Dynamic pricing
Product recommendation and ads are not the only things dynamically changing thanks to machine learning. Nowadays, most online stores and ecommerce platforms constantly adjust prices depending on the fluctuations in product demand and supply, competitors’ promotions and pricing strategies, broader sales trends, and more.
5. Chatbots
Chatbots and virtual assistants are highly interactive tools powered by machine learning and NLP and capable of providing customers with 24/7 user support (including information about available products and shipping options) while sending reminders, coupons, and personalized suggestions to upscale your sales.
6. Supply chain management
Product replenishment and other inventory management operations should never be left to chance. To better match product supply and demand, optimize space utilization in warehouses, and avoid food spoilage, it’s worth relying on the analytical and forecasting capabilities of machine learning algorithms. This means considering several variables, such as price fluctuations or seasonality-based purchase patterns, predicting future sales trends, and therefore planning proper restocking initiatives.
7. Delivery optimization
Another aspect of logistics which can be enhanced through machine learning is product delivery. ML-powered systems, fuelled with traffic and weather data collected through networks of IoT sensors and cameras, can easily calculate the fastest delivery routes. By processing user data, instead, they may recommend suitable delivery methods to better meet customers’ needs.
But the apotheosis of this approach is probably the ML-based anticipatory shipping technique implemented by Amazon, which allows to forecast future deliveries based on customers’ purchase patterns, move products to a closer warehouse, and therefore be able to dispatch them faster and inexpensively when the actual order is placed.
8. Autonomous vehicles
This embodiment of machine learning and computer vision for product delivery is still far from being perfected and implemented on a vast scale. However, companies like Amazon and Kroger are betting on this technology and soon we may rely on self-driving vehicles to speed up product distribution.
9. Video surveillance
ML-powered computer vision systems can drive vehicles… and spot thieves. The major difference between these tools and traditional video surveillance solutions is that the latter identify intruders based on a rather inaccurate rule-based approach that suffers from lots of false positives. Machine learning systems, on the other hand, can recognize more subtle behavioral patterns and alert the management if something suspicious happens.
10. Fraud detection
When it comes to online retailers and ecommerce platforms, thieves are more likely to steal money from credit cards than products from a shelf. Since machine learning algorithms are designed to identify recurring patterns, they can also pinpoint any event deviating from the norm, including anomalous transaction frequency or account data inconsistencies, and flag it as suspicious for further inspection.
Overcoming modern challenges with machine learning
Artificial intelligence, machine learning, and cognitive technologies have proven invaluable in increasing profits and optimizing costs, personalizing customer experience, enhancing operational efficiency in terms of logistics and inventory management, and ensuring a safe retail environment.
Indeed, Fortune Business Insight’s 2020 report highlighted that the global AI market in retail is expected to reach $31.18 billion by 2028, with machine learning representing its core segment.
From the retail perspective, this will make machine learning a beacon for finding the right course and docking in a safe harbor after over two years of stormy seas.
About the AuthorAndrea Di Stefano is a Technology Research Analyst at itransition.com, a Denver-based software development company. He investigates emerging tech trends and their most impactful business applications, focusing on AI, machine learning, analytics, and big data.
Featured image: ©Алексей Олейник
Share via:
7 Ways AI Will Impact Learning and Development
Natural language processing for business: a practical guide
What is AI Bias and Why It Matters to IT?